Entries from December 2011 ↓
December 19th, 2011 — Data management
…and it’s totally awesome.
Data volumes are exploding. Enterprises need better techniques to analyze, for example, IT management data or customer behavior statistics. The term ‘big data’ has emerged to describe new data management challenges posed by the growing volume, variety and velocity of data being produced by interactive applications and websites, as well as sensors, meters and other data-generating machines.

Our term ‘Total Data’ denotes a broad approach to data management that makes use of all available data, regardless of where it resides, to improve the efficiency and accuracy of business intelligence.
Total Data describes how users are deploying specialist data management technologies to maximize the benefit from individual operational or analytic workloads, while avoiding the creation of data silos by applying a unified approach to management that enables efficient data movement and integration.
This report examines the trends behind big data, as well as the new and existing technologies used to store and process this data, and outlines a Total Data management approach that is focused on selecting the most appropriate data storage and processing technology to deliver value from big data.
For more details of our Total Data report, and how to get it, see this page.
December 16th, 2011 — Collaboration
We recently published a spotlight report on cloud file sharing and sync, file backup and file-oriented collaboration in the cloud – and all the overlaps and intersections between these areas. The full report is available here for 451 Research subscribers (link requires log-in).
The idea was to shed some light on this sector that often seems to be described by its two best known players – Dropbox and Box. Despite the similar names, the services offered by these two providers have significant differences. And each is after a different, though in some cases overlapping, target market.
Dropbox in particular seems to be gaining a lot of attention from enterprise IT departments — and it’s not all good. As compliance, security, risk and IT folks in general try to get their arms around the fact that corporate data is moving to Dropbox (and other services), a number of providers have started to look at providing alternatives. All of this largely driven of course by the widespread use of iPads and other mobile devices by business users and their need to access files from these devices and keep them in sync across mobile and desktop systems. Box exploits this requirement as well, but offers more file-oriented collaboration capabilities, though not full-blown content management in the traditional sense.
Cloud file sharing, sync and mobile support for file collaboration will all be hot topics in 2012. We feel we might quickly be inundated by the number of providers that want to offer some kind of alternative to Dropbox to appease IT departments and/or better mobile access to existing enterprise content systems, like SharePoint. Below is our first-stab attempt to start to map some of this to the sub-sectors within this broader and rapidly shifting landscape. And we know it’s not comprehensive, the players here are changing almost daily.
Cloud file backup, sharing, sync and collaboration providers
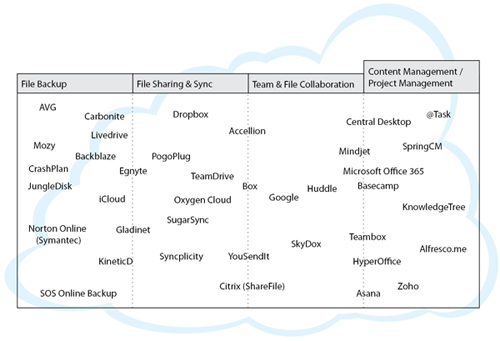
Source: 451 Research
December 16th, 2011 — Data management
The increased focus on the value of data, combined with the recent release of Moneyball, has focused much attention on Oakland Athletics general manager Billy Beane and his successful use of data to improve performance.
Beane was my no means the first to realize the potential use of data in sports, however. That title could arguably go to Valeriy Lobanovskyi, manager of the Dynamo Kyiv soccer team between 1974 and 1990.
Lobanovskyi’s name is unlikely to be well known to even the most ardent football fans but our research into Total Football as an inspiration for our total data concept has highlighted the fact that Lobanovskyi was as much a big data visionary as he was a footballing visionary.
Total football is most readily associated with Rinus Michels and his teams: Ajax of Amsterdam, Barcelona, and the Dutch national side of the 1970s; but while Michels was busy winning Dutch league titles and European Cups, Lobanovskyi similarly was busy at Dynamo Kiev winning the Soviet League eight times, the Ukrainian league five times, and the European Cup Winner’s Cup twice with an approach known as Universality.
Describing the concept of Universality, Lobanovskyi once stated that “the most important thing in football is what a player is doing on a pitch when he is not in possession of the ball.”
Total football devotees will recognize the description, and as Hortonworks co-founder Arun C Murthy recently noted, Lobanovskyi arguably deserves as much credit as Michels for coming up with what would eventually become known as total football.
So far, so football visionary. What separates Lobanovskyi from Michels is the fact that he based much of his vision on data, and the analysis of data. Originally trained as an engineer, Lobanovskyi saw the potential value of a scientific, data-led approach to sport.
Together with statistician Anatoliy Zelentsov, Lobanovskyi devised a method of recording and analyzing the events and actions in a game of football and using it to provide players with a statistical analysis of their performance and set targets designed to meet the style he wanted the team to play (squeezing, pressing, or combination).
“All life,” Lobanovskyi once said, “is a number”.
An example of Lobanovskyi and Zelentsov’s targets, as explained in Inverting the Pyramid: A History of Football Tactics, by Jonathan Wilson, is displayed below:
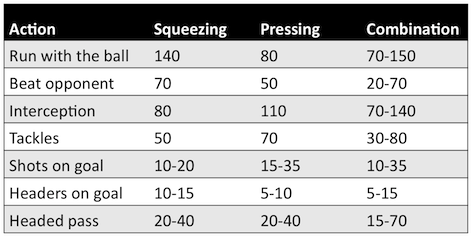
To put this in some context, Lobanovskyi was using statistics and data as a means of gaining competitive advantage in sport 20 years before the formation of Opta Sports and Prozone, and almost 30 years before Beane and the 2002 Oakland Athletics.
Clients can read more about Total Football, and our description of approaches to data management in an era of ‘big data’, in our Total Data report, to be released in the coming days.
December 14th, 2011 — Data management
In the months since we coined the term NewSQL we have come to define it as referring to a new breed of relational database products designed to meet scalability requirements of distributed architectures, or improve performance so horizontal scalability is no longer a necessity, while maintaining support for SQL and ACID.
During the recent round of NoSQL Road Show events it has emerged that this description could be taken to suggest that NewSQL products are able to provide consistency, availability and partition tolerance and therefore contravene the common understanding of CAP Theorem that “a distributed system can satisfy any two of these guarantees at the same time, but not all three.”
How is possible to provide strongly consistent distributed systems and not break CAP Theorem?
For a start, CAP Theorem is not that simple. As others have pointed out – Cloudera’s Henry Robinson for example – CAP Theorem isn’t simply a case of “consistency, availability, partition tolerance. Pick two.”
In fact the father of CAP Theorem, Dr Eric Brewer, has clarified that the “2 of 3” explanation is misleading: “First, because partitions are rare, there is little reason to forfeit C or A when the system is not partitioned. Second, the choice between C and A can occur many times within the same system at very fine granularity; not only can subsystems make different choices, but the choice can change according to the operation or even the specific data or user involved. Finally, all three properties are more continuous than binary. Availability is obviously continuous from 0 to 100 percent, but there are also many levels of consistency, and even partitions have nuances, including disagreement within the system about whether a partition exists.”
We know that CAP is not simply a case of “pick two”, since while Amazon’s Dynamo (and the many NoSQL databases it has inspired) sacrifices consistency for availability, it does so with eventual consistency, not the total absence of consistency.
Clearly is possible to have systems that are partition tolerant, highly available and offer *a degree of consistency* (although as Fred Holahan points out, whether that degree is suitable for you particular workload is another matter).
Partition tolerance is not necessarily something that can be relaxed in the same manner – in fact the proof of CAP Theorem relies on an assumption of partition tolerance. As Yammer engineer Coda Hale explains: “Partition Tolerance is mandatory in distributed systems. You cannot not choose it.”
Daniel Abadi has previously explained how CAP is not really about choosing two of three states, but about answering the question “if there is a partition, does the system give up availability or consistency?”
Just as systems that sacrifice consistency retain a degree of consistency, Daniel also makes the point that systems that give up availability also do not do so in totality, noting that “availability is only sacrificed when there is a network partition.”
As such, Daniel makes the point that the roles of consistency and availability in CAP are asymmetric, and that latency is the forgotten factor that re-balances the equation.
Daniel has also returned to the issue of the tradeoff between latency and consistency in a more recent post, noting that, unlike availability vs consistency, “the latency vs. consistency tradeoff is present even during normal operations of the system.”
The Apache Cassandra wiki actually makes this point very well:
“The CAP theorem… states that you have to pick two of Consistency, Availability, Partition tolerance: You can’t have the three at the same time and get an acceptable latency. Cassandra values Availability and Partitioning tolerance (AP). Tradeoffs between consistency and latency are tunable in Cassandra. You can get strong consistency with Cassandra (with an increased latency).”
This suggests that you can, in fact, have consistency, partition tolerance and availability at the same time, but that latency will suffer. ScaleDB’s Mike Hogan made that argument earlier this year in describing the ‘CAP event horizon’ – “the point at which latency for a clustered system exceeds that which is acceptable and then you must decide what concessions you are willing to make”.
See also Brian Bulkowski’s explanation of how Citrusleaf can claim to deliver immediate consistency by relaxing availability in the event of partition failure: “During this period, Citrusleaf will seem less highly available – that is, latencies will be higher – until the reconfiguration completes. Transactions still flow during this period – they are queued and forwarded at different places in the client and in the servers – but the cluster has, in theoretical terms, lower availability.”
Like Citrusleaf’s ACID-compliant NoSQL database, NewSQL databases are not designed to avoid the CAP event horizon by being as available as eventually consistent systems – that *would* break CAP Theorem – but arguably they are designed to delay that CAP event horizon as much as possible by delivering systems that, in the event of a partition, are highly consistent and offer *a degree of availability*.
Whether that degree of availability is suitable for your application will depend on your tolerance – not for partitions but for latency.
December 12th, 2011 — Data management
Hidden away amongst the details of our little tour around LinkedIn statistics on NoSQL and Hadoop skills was some interesting information on how many LinkedIn members list the various data management technologies in our sample in their profiles.
Our original post contained the fact that there were 9,079 LinkedIn members with “Hadoop” in their member profiles, for example, compared to 366,084 with “MySQL” in their member profiles.
Later posts showed there were 170 with “Membase” and 1,687 with “HBase”, 787 with “Apache Cassandra” and 376 with “Riak”, 6,048 with “MongoDB” and 2,152 with “Redis”, and finally, 1,844 with “CouchDB” and 268 with “Neo4j”.
This gives us an interesting perspective on the relative adoption of the various NoSQL databases:

If it wasn’t already obvious from the list above, the chart illustrates just how much more prevalent MongoDB skills are compared to the other NoSQL databases, followed by Redis, Apache CouchDB, Apache HBase and Apache Cassandra. The chart also illustrates that while HBase is the second most prevalent NoSQL skill set in the USA, it is only fourth overall given its lower prevalence in the rest of the world.
In response, a representative from a certain vendor notes “Some skills are more valued not because they are more prevalent, but because they are harder to achieve.” Make of that what you will.
December 9th, 2011 — Data management
Following last week’s post putting the geographic distribution of Hadoop skills, based on a search of LinkedIn members, in context, this week we will be publishing a series of posts looking in detail at the various NoSQL projects.
The posts examine the geographic spread of LinkedIn members citing a specific NoSQL database in their member profiles, as of December 1, and provides an interesting illustration of the state of adoption for each.
We’ve already taken a look at Membase and HBase; Apache Cassandra and Riak; and 10gen’s MongoDB and Redis.
Part four brings the series to a close with a look at Apache CouchDB and Neo4j, which boast the most geographically diverse adoption of the NoSQL databases in our sample.
The statistics showed that 36.4% of the 1,844 LinkedIn members with “CouchDB” in their member profiles are based in the US, while only 8.9% are in the Bay area, the least of any of the NoSQL database we looked at.
The results also indicate that the UK is a particularly strong area for CouchDB skills, with 7.1%. Other hot-spots include Canada (4.1%), Germany (4.0%) and The Netherlands (3.1%).
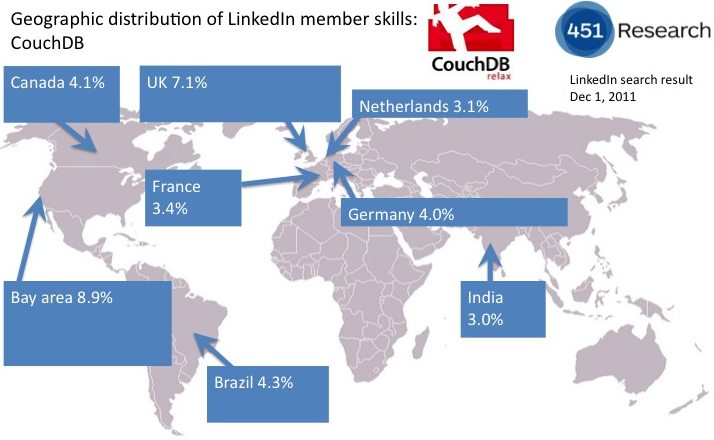
Neo4j is even more widely adopted, with only 36.2% of the 268 LinkedIn members with “Neo4j” in their member profiles based in the US, although 10.4% are in the Bay area.
With 4.1%, Sweden is a hot-spot for Neo4j skills, as one might expect given that’s where it and Neo Technology originated. The UK is also strong with 9.7%, followed by India with 5.6% and the New York area with 4.9%.
Since Neo4j originated in Europe it is of course an open question whether its higher adoption in the Rest of the World than the US is a sign of a greater spread of adoption, or a relative failure to infiltrate the US market. Given that the company already has an active presence in the US we are inclined towards the former.

N.B. The size of the boxes is in proportion to the search result (click each image for a larger version). World map image: Owen Blacker
December 8th, 2011 — Data management
Following last week’s post putting the geographic distribution of Hadoop skills, based on a search of LinkedIn members, in context, this week we will be publishing a series of posts looking in detail at the various NoSQL projects.
The posts examine the geographic spread of LinkedIn members citing a specific NoSQL database in their member profiles, as of December 1, and provides an interesting illustration of the state of adoption for each.
We’ve already taken a look at Membase and HBase, and Apache Cassandra and Riak. Part three examines the geographic spread of 10gen’s MongoDB and Redis.
The statistics showed that 41.0% of the 6,048 LinkedIn members with “MongoDB” in their member profiles are based in the US, putting MongoDB is the top half of the table for geographic spread.
Only 11.2% are in the Bay area, fewer than Hadoop, Membase, HBase, Cassandra, Riak and Redis. The results also indicate that the New York area is a hot-spot for MongoDB skills, with 6.2% – as one might expect given the location of 10gen’s HQ. Other hot-spots include Brazil (4.2%) and Ukraine (2.8%).
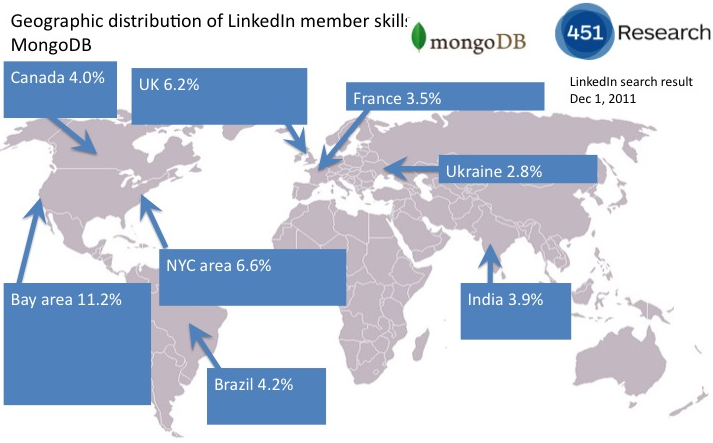
Redis is even more widely adopted, with only 37% of the 2,152 LinkedIn members with “Redis” in their member profiles are based in the US, although 12.0% are in the Bay area.
Ukraine is also a hot-spot for Redis skills (3.8%) as is France (3.6%) and Spain (2.9%).

The series will conclude later this week with CouchDB, and Neo4j.
N.B. The size of the boxes is in proportion to the search result (click each image for a larger version). World map image: Owen Blacker
December 7th, 2011 — Data management
Cloud computing and big data are two of the hottest topics in the industry today, which makes cloud databases a particularly hot prospect for 2012. What is a cloud database, however? On Thursday, December 15 at 12:00pm EST I’ll be taking part in a webinar with Karen Tegan Padir, Vice President of Products and Marketing, EnterpriseDB on the subject of cloud computing and true cloud databases.
In this webcast, you’ll get an overview of the current state of cloud database computing, and more specifically the differences between cloud databases and databases in the cloud. I’ll be providing an overview of the functional requirements that separate databases running in the public cloud, and databases that will be used to power private and hybrid clouds.
Then Karen will provide an overview and demonstration of Postgres Plus Cloud Server, which provides DaaS for PostgreSQL databases and went into public beta earlier this week.
You can register for the event here
December 6th, 2011 — Data management
Following last week’s post putting the geographic distribution of Hadoop skills, based on a search of LinkedIn members, in context, this week we will be publishing a series of posts looking in detail at the various NoSQL projects.
The posts examine the geographic spread of LinkedIn members citing a specific NoSQL database in their member profiles, as of December 1, and provides an interesting illustration of the state of adoption for each.
Following yesterday’s look at Membase and HBase, part two examines the geographic spread of Apache Cassandra and Basho Technologies’ Riak.
The statistics showed that 52.2% of the 787 LinkedIn members with “Apache Cassandra” in their member profiles are based in the US (as previously explained, we had to use the ‘Apache’ qualifier with Cassandra to filer out people with the name Cassandra).
A significant proportion (18.0%) of those are in the Bay area, although fewer than Hadoop, Membase and HBase. The results also indicate that Canada is a hot-spot for Apache Cassandra skills, with 4.1%, while Apache Cassandra is also making in-roads into Europe via France and Spain.
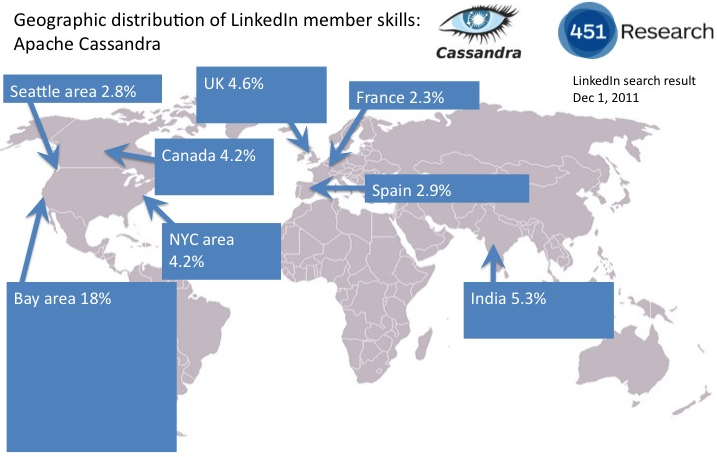
Basho’s Riak is less dependent on the USA for adoption. The statistics showed that less than half – 45.5% – of the 376 LinkedIn members with “Riak” in their member profiles are based in the US, with only 13.0% in the Bay area.
Riak hot-spots include the UK (6.9%) and Australia (4.3%). as well as the Boston area, in keeping with the company’s HQ.

The series will continue later this week with MongoDB, CouchDB, Neo4j, and Redis.
N.B. The size of the boxes is in proportion to the search result (click each image for a larger version). World map image: Owen Blacker
December 5th, 2011 — Data management
Following last week’s post putting the geographic distribution of Hadoop skills, based on a search of LinkedIn members, in context, this week we will be publishing a series of posts looking in detail at the various NoSQL projects.
The posts examine the geographic spread of LinkedIn members citing a specific NoSQL database in their member profiles, as of December 1, and provides an interesting illustration of the state of adoption for each.
We begin this week’s series with Membase and HBase, the two projects that proved, like Apache Hadoop, to have significantly greater adoption in the USA compared to the rest of the world.
The statistics showed that 58.2% of the 170 LinkedIn members with “Membase” in their member profiles are based in the US (as previously explained, we tried the same search with Couchbase, but with only 85 results we decided to use the Membase result set as it was more statistically relevant).
As with Hadoop, a significant proportion (27.1%) of those are in the Bay area, the highest proportion of all the NoSQL databases we looked at. The results also indicate that Ukraine is a hot-spot for Membase skills, with 3.5%, while Membase adoption is lower the UK (2.4%) than other NoSQL databases.

It should not be a great surprise that Apache HBase returned similar results to Apache Hadoop. The top eight individual regions for HBase were exactly the same as for Hadoop, although the UK (3.4%) is stronger for HBase, as is India (10.7%).
The statistics showed that 57.0% of the 1,687 LinkedIn members with “HBase” in their member profiles are based in the US, with 25.0% in the Bay area (the third highest in our sample behind Hadoop and Membase).
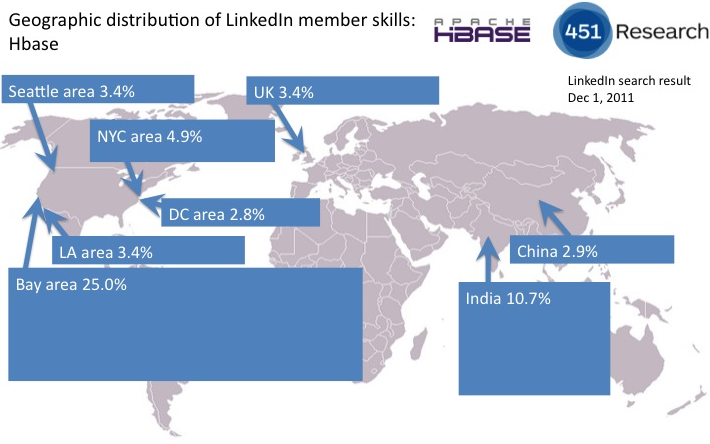
The series will continue later this week with MongoDB, Riak, CouchDB, Apache Cassandra, Neo4j, and Redis.
N.B. The size of the boxes is in proportion to the search result (click each image for a larger version). World map image: Owen Blacker